· 3 min read
Trust But Verify - How to Consider Claims by Legal AI Vendors
273 Ventures CEO, Michael Bommarito, Quoted in LegalTech News Discussing Exaggerated Claims by Legal AI Vendors
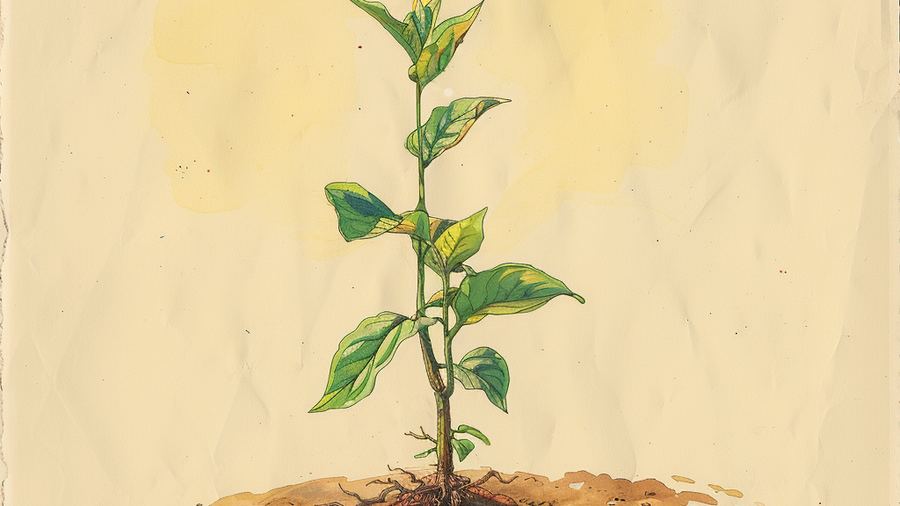
In a recent article by LegalTech News, Michael Bommarito, CEO of 273 Ventures, was quoted on a growing concern in the legal tech world: the murky waters of AI jargon. Bommarito pointed out, “I’ve seen an increase in the number of people who are taking something that one of the other [AI] vendors upstream has built and making it sound like they’ve done anything close to a material amount of work or innovation or unique enterprise value creation and actively attempting to confuse the buyer.” This quote cuts to the heart of the issue — there’s a lot of noise out there, and it’s making it tough for legal professionals to understand what’s what in the world of AI.
As is noted in the article, when it comes to developing AI models, there’s a big difference between “training” and “fine-tuning.” Training an AI model is like growing a tree from a seedling with careful curation of the soil, water, and access to sunlight. But here’s the kicker: not many in the legal tech space are actually doing this. It’s a massive task requiring tons of resources, time, and expertise. That’s why Bommarito calls out those who claim to have trained a model from scratch (when in fact they have not) as being “somewhere between negligent in your marketing and purposely dishonest.”
On the flip side, “fine-tuning” is the more go-to method for most legal tech companies. This is where you take an existing AI model — akin to selecting a sapling with its inherent characteristics and potential limitations — and teach it new tricks that are specific to your needs. For example, you might grab a model like Google’s Llama and tweak it to better understand legal documents. It’s a practical way to get AI that works for you without starting from zero.
Beyond training and fine-tuning, there are also fancy tricks like prompt engineering and retrieval-augmented generation (RAG) that tweak how an AI model responds to questions or searches for information. Prompt engineering is all about asking the right questions to get the best answers, while RAG helps the model pull in extra info from outside sources to beef up its responses.
Importantly, fine-tuning, prompt engineering and RAG cannot always compensate for the original shortcomings of the underlying model. Just as a sapling’s future health and growth are highly dependent on its genetic makeup and pre-existing conditions, an AI model’s effectiveness is potentially limited by its initial design and training data. A model built from first principles is highly likely to do better than a model that you have to resuscitate after the fact.
Understanding these differences isn’t just academic; it’s crucial for anyone in the legal field looking to make smart choices about AI. It’s not just about cutting through the jargon; it’s about building a foundation of trust and transparency between AI vendors and the legal professionals they aim to serve.
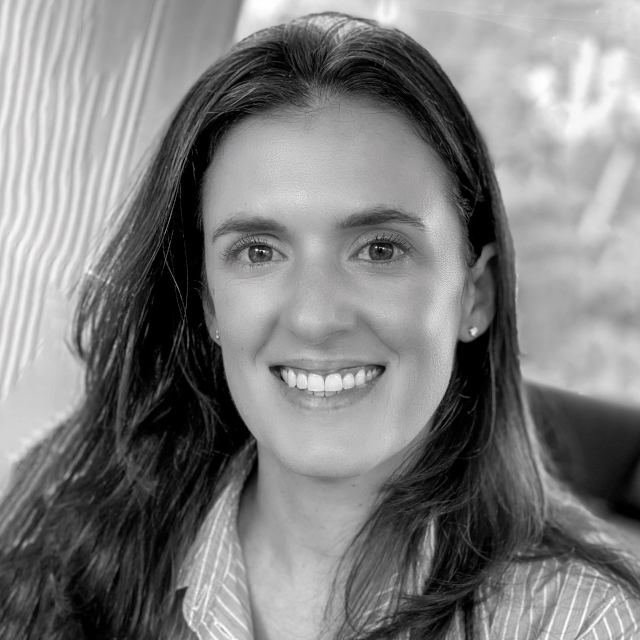
Jessica Mefford Katz, PhD
Jessica is a Co-Founding Partner and a Vice President at 273 Ventures.
Jessica holds a Ph.D. in Analytic Philosophy and applies the formal logic and rigorous frameworks of the field to technology and data science. She is passionate about assisting teams and individuals in leveraging data and technology to more accurately inform decision-making.
Would you like to learn more about the AI-enabled future of legal work? Send your questions to Jessica by email or LinkedIn.